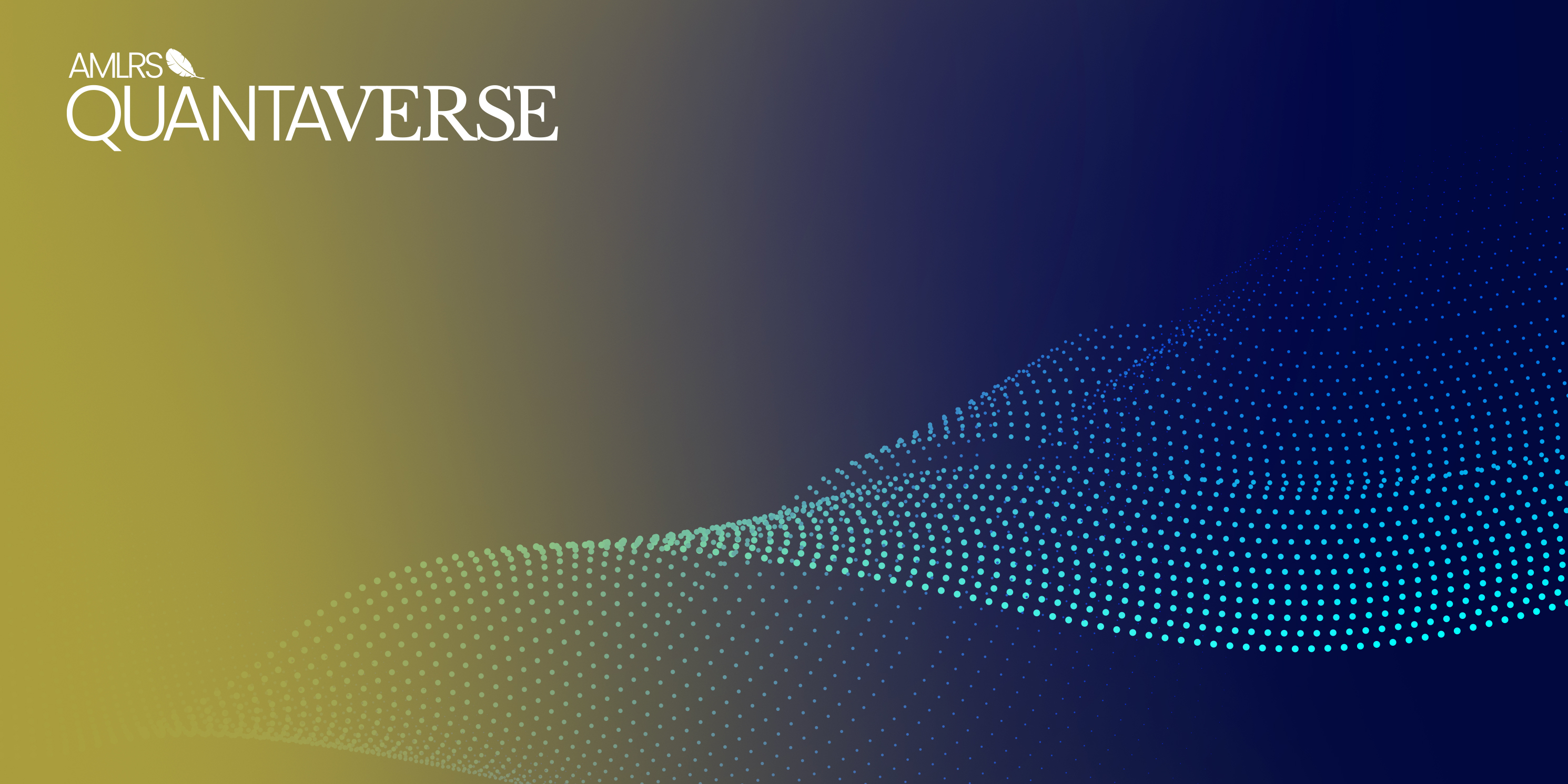
Financial institutions face a double whammy when it comes to anti-money laundering (AML) compliance. On one hand, they must consistently review and detect suspicious activity in an ever-growing stream of transactions. At the same time, compliance teams must meet that standard with whatever resources and support they are offered and avoid compliance fines by meeting regulatory expectations.
The risks of fines run higher than many may think. In December 2021, a UK-based bank was fined $85 million by regulators for AML process failures spanning eight years. The Financial Conduct Authority (FCA) identified serious weaknesses in three key parts of the bank’s transaction monitoring system (TMS) in Britain from 2010 to 2018. In the same year, the UK-based bank’s rival was also fined $350.9 million for failing to prevent the laundering of nearly 400 million pounds, some of it deposited at a branch in bin bags.
Despite serious implications, many financial institutions are still fighting money laundering with as minimal resources as possible. As a result, the risk of regulatory penalties remains high. Artificial intelligence (AI) and machine learning hold the key to helping institutions efficiently reduce regulatory risk and improve the AML investigation process despite resource restrictions.
Applying AI to AML Compliance to Reduce Risk
A key reason that the risk of regulatory compliance fines remains high while so much crime still goes undetected is that most companies are still relying solely on legacy TMS. A standalone TMS is a liability for two reasons:
- The high percentage of crime or risk that TMS miss because a transaction does not violate a programmed rule. The United Nations Office on Drugs and Crime (UNODC) estimates that the amount of money laundered globally in one year is two to five percent of global GDP.
- The number of false positives a TMS creates monopolizes the valuable time and attention of human investigators. The industry estimates that approximately 95% of the alerts generated by transaction monitoring systems are false positives.
Artificial intelligence is uniquely suited to address these challenges.
Applications powered by AI are developed to enhance human cognitive performance or take over some tasks by enabling machines to emulate human intelligence processes, including:
- Learning: gathering information and acquiring the rules needed to use that information
- Reasoning: drawing conclusions, and
- Self-correction: improving future outcomes based on feedback to past reasoning.
Modern AI systems have progressed to the point where large volumes of transactional and other sources of data can be culled, consolidated, analyzed, and scored for risk, so that investigators can make more accurate determinations. This makes the application of AI technology a logical solution to alleviating the three critical AML compliance challenges financial institutions – reducing false positives, automating steps of the investigation process, and discovering the risk that current systems miss.
Artificial intelligence improves AML compliance by identifying patterns and discerning anomalies that traditional approaches cannot fully resolve and which current systems regularly miss. It can efficiently discern truly nefarious alerts and catch the false negatives that embody both financial and reputational risk.
QuantaVerse AI is Born for AML Compliance
AML RightSource’s QuantaVerse Platform is the industry-leading AI and machine learning platform for identifying and investigating financial crimes. Validated, proven, and easy to deploy, the QuantaVerse Platform is purpose-built to help AML compliance teams improve their programs and protect against regulatory compliance fines.
Working alongside a rules-based TMS, the AI system in the QuantaVerse Platform can go deeper and broader to better detect patterns of behavior, analyze the intent of those patterns, and expose anomalous activities.
Transactions that do not follow the usual frequency and directional patterns expected for a given type of account may not be flagged by a TMS. However, they would be identified by the QuantaVerse Platform. For example, the QuantaVerse Platform learns the baseline of normal reported payroll account activity by line-of-business and identifies irregularities in payroll transactions worthy of further investigation.
The QuantaVerse Platform’s machine learning capability then leverages the observables collected and assigns risk scores to each. These scores embody the level of suspicion around transactions, transacting entities, and entity networks providing a holistic view of transacting entities and their motives by representing the “what,” “who,” and “why” of financial activities.
Risk scores are accompanied by a measure of confidence about the risk assessment. Confidence is calculated based on variables including the number of alerts and anomalies detected, as well as similarity to past cases. This allows the AI system in the QuantaVerse Platform to constantly evolve, learning from past decisions.
The advanced machine learning capabilities of the QuantaVerse Platform also enable it to identify new money laundering techniques and trends way ahead of any legacy system and tips off investigative teams to new behaviors. Feedback provided by the platform also helps better refine TMS rules.
Specific AI and machine learning techniques that the QuantaVerse Platform employs to identify transactional anomalies for further investigation include:
- Collaborative filtering: capable of finding transactions with missing, matching and/or odd information
- Feature matching: utilized to identify transactions above and below a specific monetary threshold
- Fuzzy logic: used to find data matches with slight changes to data such as names or addresses
- Cluster analysis: detects abnormalities in transactions benefiting a single person or entity
- Time series analysis: identifying transactions benefiting a person or entity over an extended period
- Focused keyword searches: dynamically monitors, screens, and filters transactions based on keywords from high-risk AML, CTF, and financial crimes typologies
- Pattern detection: learns from an AI-identified suspicious activity to enhance transaction monitoring and KYC platforms and programs
Act Now to Prevent Regulatory Compliance Fines
In the field of AML, AI and machine learning help prevent situations that could result in regulatory compliance fines. For financial institutions, the time has come to deploy AI and machine learning into their AML ecosystems. Holding the key to reducing risk related to financial crimes, these technologies address regulation, drive out operational costs through improved efficiency and, most importantly, effectively prevent criminals and terrorists from using the banking industry for their illicit agendas.