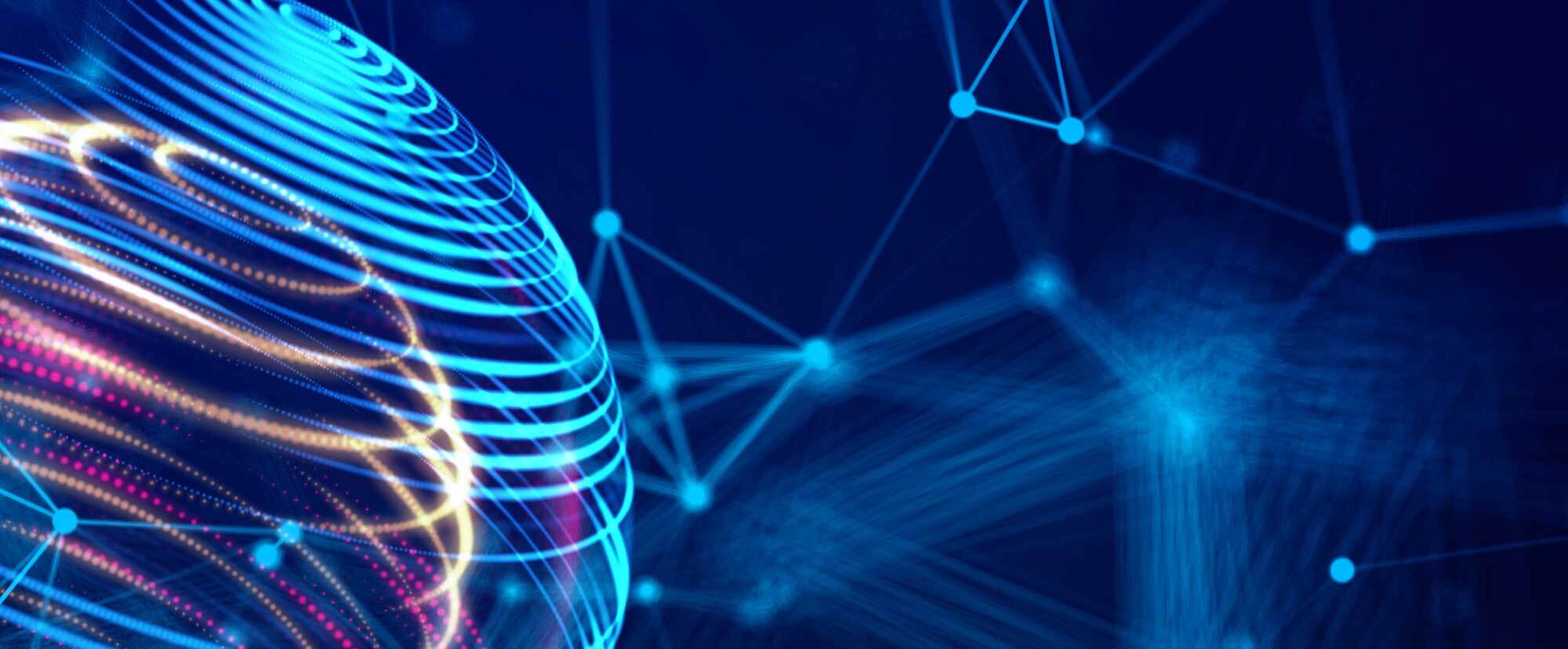
In highly regulated industries, the need for enhanced due diligence (EDD) on customers and counterparties has never been so necessary. In the case of tier one banks and other large financial institutions (FIs), compliance protocols can differ between jurisdictions managed by national regulatory bodies, which makes adherence all the more difficult.
However, what can string together diversified regulatory checks is the recommended leveraging of adverse media screening. It is a universal trend for organizations that wish to conduct thorough due diligence checks, and stay in line with ever more stringent AML rules.
Defining Adverse Media
Adverse media screening is an important cog in the whole AML process as it can cover a wide range of use cases for FIs. For instance, during the initial onboarding stage of an individual or company for basic KYC checks, periodic reviews, and the analysis of transaction monitoring alerts.
However, adverse media is a tough area to define despite its global recommendations. It can consist of a range of data points from a variety of data sources: news websites; regulatory documents; social media posts and blog articles; newsletters and pamphlets; and many more. All keywords that stem from an adverse media search can be useful to flag areas of risk and the need for further AML investigations.
The Growing Need
The sporadic nature of adverse media sources – especially considering its format as being either structured or unstructured data – causes numerous concerns for financial investigators. With a vast spectrum of data points, the threat of duplicate results and a large number of false positives can lead to cumbersome and inefficient investigative practices, especially when adding human error into the mix.
Many investigators could use Google for compliance searches which, while a popular source of information for a casual surfer, lacks the necessary requirements for investigators at FIs that need specific keywords from valued sources to conduct advanced AML checks. Given the rise of fake news in recent years, dubious news sources can be mixed with all results from a Google search, meaning that the already hefty ‘noise’ from a data search is exacerbated. The focus on high-risk entities can be skewed with a large influx of false positives.
Machine Learning Solutions
Multiple countries are looking to adopt adverse media screening to combat any inadequate AML protocols through the moves of their respective regulators. In the United States, FIs are obliged to comply with the Bank Secrecy Act (BSA) as outlined by the Financial Crimes Enforcement Network (FinCEN) – adverse media screening can assist data gathering for, say, the filing of SARs. Elsewhere, the Sixth Anti-Money Laundering Directive (6AMLD) in the EU requires the screening of high-risk flags in cross-jurisdictional financial crime activity, while adverse media screening has been recommended in the APAC region to tighten AML procedures following the 1MBD scandal in 2018. All this can be assisted through machine learning.
Adverse media screening for these aforementioned purposes can generate a whole range of fraud alerts, involving a huge workload for human investigators. Machine learning looks to automatically process false positives in order for the most prevalent flags of high-risk entities to be assigned to skilled AML teams for further investigation. Plus, when both structured and unstructured data sets are concerned, through the use of machine learning models these different threads of information can all be amalgamated to create a consolidated view of an entity. Transaction histories and personal background information (as flagged by relevant adverse media searches) become building blocks towards a holistic picture of an individual or organization. Indeed, their relationships with other third parties can also be mapped through analysis of strange behavioral patterns identified by machine learning techniques.
Adverse media screening can become an integral part of client onboarding, and throughout ongoing reviews in an AML investigation for FIs. From discovering the most relevant high-risk fraud alerts through machine learning techniques, then passing through the most reliable flags to human investigators, this hybrid approach optimizes the efficiency of AML processes. Not only does this please the regulators, but it also saves costs and ensures happier customers for FIs moving forward.