4 min read
Client monitoring: reducing false positives and leveraging STP
AML RightSource
:
April 12, 2021
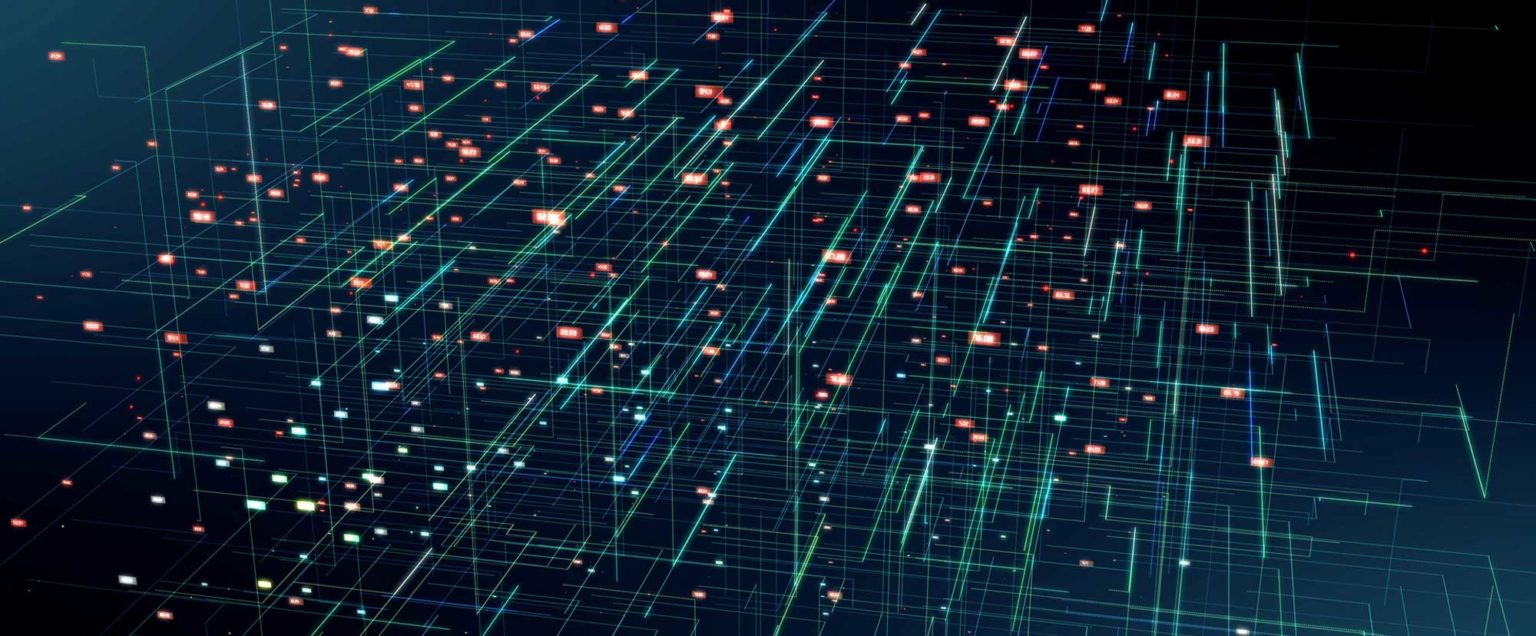
Despite the massive cost and reputational risks Financial Institutions (FI) are exposed to, efficient fraud & AML systems largely remain elusive. Furthermore, incumbent fraud systems generate a large volume of false positives in the process of detection, where a false positive can be an account or transaction mistakenly identified as criminal in origin for example. Typically, such fraud systems are over-reliant on large numbers of human fraud case handlers to course correct. This not only incurs a large cost to the FI, but it also negatively impacts the overall resilience of the fraud system by ensuring the poor allocation of resources.
Human investigators have to spend the majority of their time clearing false positives instead of focusing on complex, higher risk cases that are suited to human intervention. What should happen is the bulk of genuine transactions or accounts are correctly labelled and remain unimpeded, where only those at elevated risk status are assigned for human investigation. To achieve this state, a much more precise fraud detection system is required and reducing false positives remains a key challenge.
Fincrime challenges
The financial crime domain has always been a challenging one to work in. At its core, the problem can be posed as trying to contain the creativity and ingenuity of humans, which is what makes it so difficult to develop a robust fraud system. Technology obviously plays a key role but so does the human element. Criminals will relentlessly prod and probe systems until they find a weakness, then exploit it for maximum gain until the attack vector is compromised. Criminals know banks can’t label everything as fraud and manually investigate, so they are always trying to find the gaps in the system that won’t get flagged as such.
An example could be criminals discovering two mule accounts between different banks, and a transaction value of £4999 is enough to rapidly move money without setting off any fraud detection systems. Once identified, this route will be used repeatedly by criminals until it is caught and shut-down by a financial crime investigation. Criminals will then try and find other methods of attack to circumvent fraud systems and the process begins again. One of the biggest challenges for combating fraud and AML is that financial institutions approach the problem from a transaction monitoring perspective. In the example above, both banks will have a limited knowledge of accounts beyond their own transactional boundary, especially if they are dormant mule accounts with very little history, making it easy to see why the accounts or payments wouldn’t necessarily flag as fraudulent.
The client monitoring solution
If we could shift from monitoring transactions to client monitoring to tackle the problem, we can leverage a more complete context behind actions to form a decision. These decisions also aren’t as limited by the lack of transactional knowledge across the boundaries of individual financial institutions. It essentially gives a more holistic view around who or what entity you are doing business with and the risk that comes with it. If we could approach the world of fraud from a client monitoring perspective then, we could reduce the number of false positives and develop more robust fraud and AML systems in the process. Given the huge advances made in recent years in the realm of data and decision science, we are well positioned to deliver the fraud and AML systems of the future.
To shift from transaction monitoring to client monitoring is a non-trivial undertaking. It requires a huge amount of data processing and analysis for each customer, which should be continuously updated or monitored over time. In theory, it should combine transactional history (if available) and any background information for that entity, such as relevant adverse media hits. This would give a truly holistic view of each client and allows a much better understanding of possible risk exposure to the FI.
Advanced machine learning
From this much improved data landscape we can leverage data analytics and advanced machine learning techniques to identify unusual or risky patterns of behaviour. This is where the real power of data science can be seen. There are numerous machine learning techniques and approaches available for pattern recognition toward this problem area, the key is framing the problem in the right way with good underlying data. Regardless of methodology, we are trying to find a way to map the input client information to an output notion of risk. A mapping can be made for any process that fraud systems generate alerts for, such as onboarding an overseas client at the KYC stage or unusual account opening activity by a young customer. In the old world, a human fraud handler would have to investigate these alerts manually and come to a decision. As discussed, lots of these alerts are usually false positives and non-fraudulent in nature, taking up valuable resources.
In the new world where we have a more complete client profile and machine learning models to analyse alerts raised by a fraud detection system, we can process immediately by developing a suitable risk framework to decide which alerts require human intervention. Alerts that are very low risk in nature can be straight through processed (STP) by a relevant machine learning model, whereas medium to high risk alerts will always require a human in the loop to investigate. Given the skewed nature of false fraud alerts, most will be low level risk in nature and by automatically processing them we can remove the bulk of the task from humans. Typical fraud alerts number in the thousands or tens of thousands a day, so the reduction of workload will be very significant. This shift of resource allocation and the inherent scalability of machine learning models is what makes it so powerful.
Furthermore, we can utilise the machine learning models to assist human investigators by providing the analysed information for any elevated case. This supporting information could be anything from a list of detected adverse media prioritised by risk and subject matter, to a breakdown of anomalous account activity that looks outside the norm for the respective client given historical behaviours. With the right data underneath and machine learning models bulk processing fraud alerts, auto-generating supporting analyses for financial crime investigations should become routine.
Of course, achieving this state will require perpetual client monitoring, and this comes with its own distinct challenges. For instance, we will need to correctly identify and match names across disparate data sources and different jurisdictions. Intelligent processing of media will also be required to correctly identify positive or negative attributes in relation to certain topics and entities. This will rely on advanced machine learning models, specifically in the Natural Language Processing domain, to be realised. All of this information will then need to be updated at regular intervals for each known entity, so a current view of clients is obtainable. It is a truly massive data task but one that must be achieved to fully leverage the capabilities offered by client monitoring and straight through processing of false positives. This will help deliver us the next generation of AML and fraud systems.