3 min read
Harnessing Machine Learning and RPA to Automate Financial Crime Investigations
AML RightSource
:
January 10, 2021
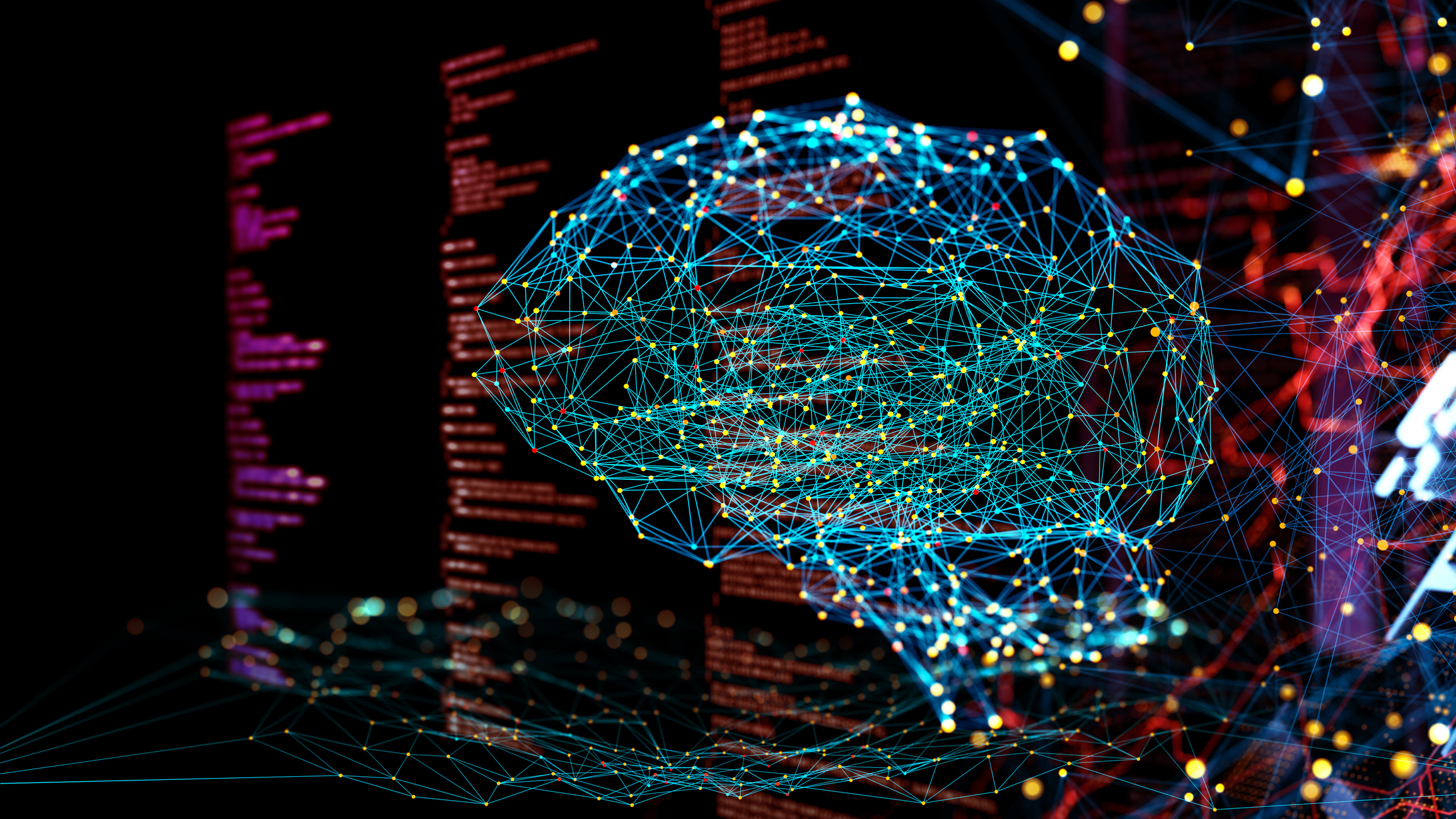
Disruptive technologies are no longer the stuff of science fiction. They’re not even the “next big thing.” Successful firms are using them right now to automate manual processes in their AML programs.
According to accounting giant PWC, 45% of work activities can be automated. Robotic process automation (RPA) is one of the technologies making this possible. As the name implies, RPA technology uses software robots, or “bots,” to perform time-consuming, rules-based office tasks. It reduces cycle time and at lower costs than other automation solutions.
Many financial firms are using RPA to find data for investigators. RPA bots are replacing manual processes by gathering data from multiple sources. This helps solve the “swivel-chair” problem experienced by many compliance professionals.
That, however, is as far as the efficiency gains of RPA go. The technology does an excellent job of collecting data for investigators, but it is unable to analyze that collected data and select the data that is relevant to the investigation. While automated data collection is an important time saver, with RPA-only solutions, time-strapped AML compliance teams are still in the position of having to sort through, select and analyze the data to make sense of it.
For example, RPA can automate the discovery of a piece of adverse media. It can serve that news article up to an investigator. However, it is unable to discern if the entity mentioned in the article is a good, bad, or neutral actor. An investigator is still required to analyze the article to determine its relevance to the investigation.
The efficiencies afforded by RPA are the first step towards AML automation success. RPA creates the foundation for machine learning — a game-changer driving improved effectiveness and efficiency within AML compliance.
The Power of Machine Learning
Machine learning picks up where RPA leaves off. Without programming, it learns and improves from experience. It analyzes huge data sets, identifies patterns, and pinpoints where exceptions or anomalies exist.
Used in conjunction with RPA, machine learning automates a significant portion of the AML investigation process by:
- Functioning in repeatable, scalable processes that lower the operations risk inherent to AML compliance teams and processes
- Reducing false positives from transaction monitoring systems
- Streamlining the selection of data that is relevant to each investigation
- Risk-scoring entities within the context of an organizations unique risk appetite
- Logically and consistently presenting analyzed data for investigator adjudication
- Auto-generating narratives to speed reporting of cleared cases and SARs in a consistent manner
- Decreasing risk by finding financial crimes that current processes miss
For example, machine learning can automate the analysis of adverse media. It can determine a new article’s context and identify whether the entity mentioned is a bad actor or not. And when assisted by natural language processing (NLP), it can:
- Report why transactions or entities seem suspicious
- Explain the significance of that suspicious activity
This automated analysis streamlines a laborious and time-consuming aspect of the AML investigation. It provides investigators with accurate and relevant information, and dramatically improves their decision-making capabilities.
Automate Your Firm’s AML Compliance Program
Financial institutions can navigate risk and compliance while optimizing their AML operations in any of the following ways:
- Build a solution in-house – a viable strategy for firms with access to a top team of data scientists capable of building and maintaining their AML technology.
- Hire a consultant – a workable approach for firms with big budgets. The cost of hiring a consultant and their subcontracted data scientist is high. System maintenance requires frequent re-engagement or the onboarding of a data science expert.
- Combine multiple point solutions – a practical method to address individual AML tasks such as entity resolution and adverse media screening. However, the implementation of two or three independent solutions still leaves large productivity gaps. Unforeseen conflict between point solutions can also create new, unpredictable issues.
- Install a packaged solution – an easy-to-deploy solution can be operational within weeks. Purpose-built for AML, a packaged solution marries the data-gathering strengths of RPA with the analytical capabilities of machine learning. The solution also receives continuous improvements based on the experience and needs of all the financial institutions it serves.
Optimize the efficiency and effectiveness of your firm’s AML program
No matter how you do it, the benefits of automating your firm’s investigative process are clear. RPA and machine learning improve efficiencies and reduce costs. The day-to-day work of investigators becomes more rewarding as they spend more time on complex and high-risk cases. Automation also improves adjudication and reporting consistencies. The result? Better compliance and far less risk for your organization.
Validated and tested in real-world environments, the QuantaVerse Financial Crime Platform proves its value to AML teams and regulators alike. The easy-to-implement packaged solution uses:
- RPA bots to source and organize data
- Machine learning tools to automate the investigation and entity resolution
- A Financial Crime Investigation Report (FCIR) to deliver recommendations and supporting data to investigators
To learn more about automating your firm’s financial crime investigation program, please visit: QuantaVerse.net/our-solutions.