5 min read
How 8 Technology Megatrends are Shaping the Future of AML Compliance
AML RightSource
:
January 31, 2022
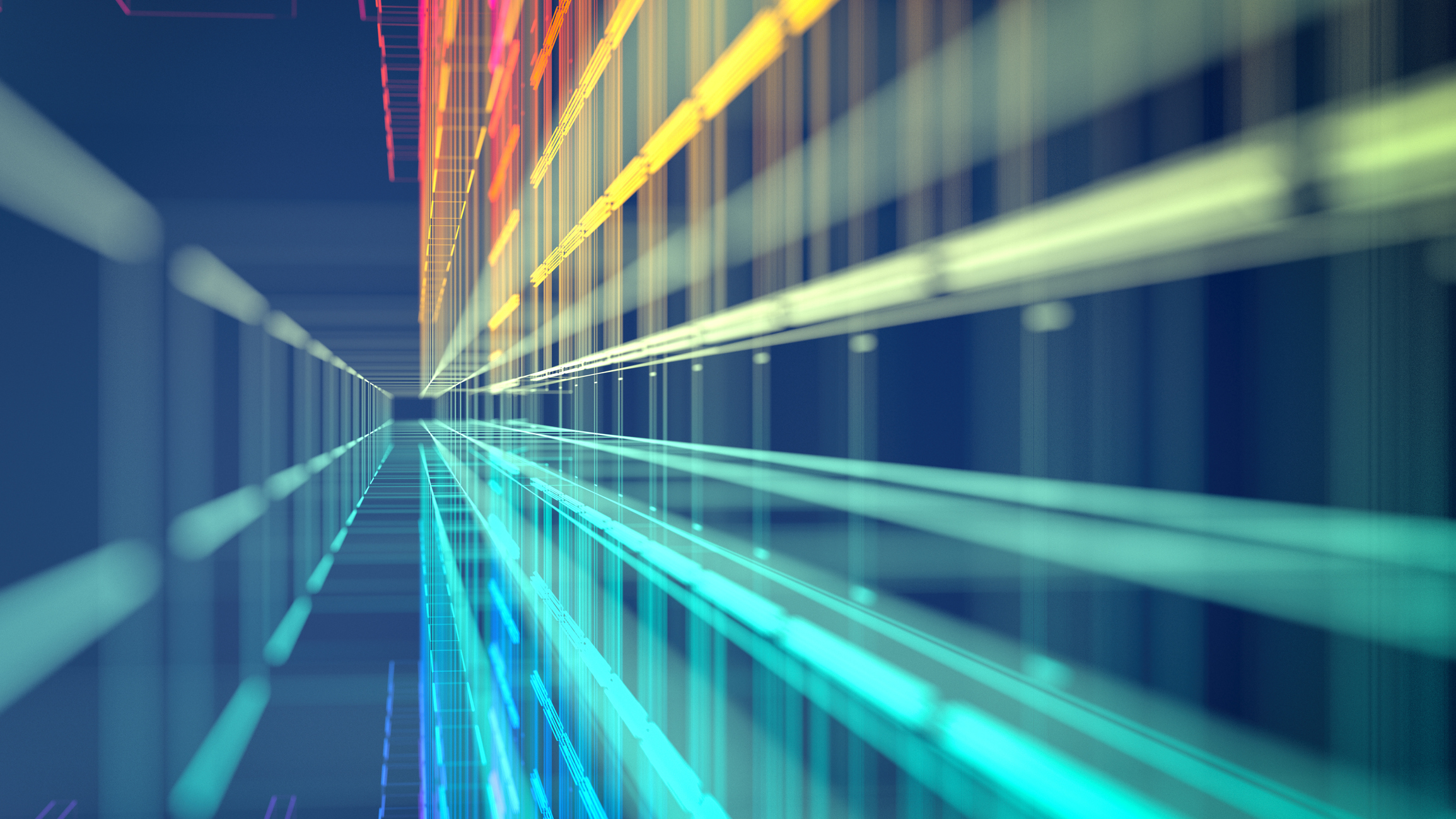
With an eye on what’s next for AML, we recently connected with Ken Harvey, former COO/CIO of HSBC Holdings, who outlined eight technology megatrends that are impacting AML compliance. We also look at how AML compliance technology is being developed, advanced, and applied to the compliance world.
-
Big Data and AI are the Critical Technologies Influencing AML Compliance Technology
Due to a steep increase in the number of active criminals, the volume of egregious types of financial transactions is high. The purchase of equities, payments, or foreign transactions are all running at record levels and growing in a more than linear fashion. This huge volume of growth in all categories results in a self-defining big data problem. Financial Institutions are faced with having to find needle-like transactions in a massive data haystack.
Another technology, which will be absolutely critical to AML efforts going forward, is artificial intelligence (AI). Financial institutions need AI to deal with the increasing volume of transactions in a way that meets ever-rising expectations that they operate without letting false positives slow things down. Financial institutions don’t want to annoy their good customers with delays. They don’t want to increase their operating expenses. Rather, they need AI to dig into this data in a way that they haven’t in the past, to reduce the number of false positives, and to find the bad guys who have historically slipped through the cracks.
-
Legacy Systems are No Longer Effective and Need to be Supplemented or Replaced
Historically, most AML systems are transaction management systems (TMS). They’re very old systems, generally built-in COBOL, which means they require a fair amount of renovation due to the programming language they use. However, their biggest drawback is that they deal with formatted data, and they analyze it with a formatted process. This is a flawed technique. It makes it impossible to catch anything outside the formatted data. A lot of effort is required to format the data to get it in all the right buckets before all the alerts are dealt with by human investigators.
An ever-growing problem with TMS, in general, is that these systems require many investigators to research false positives, and to look into other avenues or vectors of data. They must determine how long the financial institution has known the customer, if the transaction is within pattern, and so on. Staffing challenges brought about by COVID-19 made many financial institutions realize how severe the problems of these very old systems are.
Out-of-date TMS technology needs to be replaced. However, they should not be replaced with technology that uses large formatted, structured transactions with structured lists, simply because it requires too much human effort to manage.
-
Knowing Your Customer Requires Understanding Your Customer's Patterns
Going forward, knowing your customer is not going to be about simply confirming the identity and address of new customers, but rather about how that customer has behaved over time. Financial institutions will have to establish a recognition of a pattern and the development of a process to test the validity of a transaction or if the customer’s behavior is something the institution would approve of.
Payment processors are going to have to catch up with credit card companies that have become quite adept at catching fraud. Knowing your customer is about pattern recognition, pattern management, and monitoring the exceptions. However, unlike credit card companies, who deal with formatted data, payment processors will have to deal with much larger unformatted data collections from many more sources to determine if a customer is transacting in a particular pattern.
-
The Adoption of New Technologies is Going to Increase Rapidly
Quicker adoption of new technologies will be driven by the penalties for getting it wrong and by the fact that the technologies are mature and proven. They can scale. The technological barrier that normally slows adoption rates is no longer there.
The cost barrier to getting into these new technologies is also no longer an issue. It’s inexpensive to store and process large amounts of data - especially with the proliferation of cloud-based services. A lot of scale is expected to go to the cloud. It is already possible to run huge amounts of transient data through pattern recognition or AI algorithms to determine what is good and what is bad. The cloud-based throughput is there, the scale is there, as are the programming languages that can run everything fast.
Now, financial institutions need to search for the most effective algorithms and solutions and start running transactions through them. This replaces the current cost of running data through a big TMS and having hundreds of human investigators examine the matches that come out of the system. With no cost barriers and no problems of scale, the adoption of the new technologies is going to be more revolutionary than evolutionary.
-
Whoever is Last to the Party in Terms of Adopting New Technologies Will Lose Their Competitive Edge
When it comes to AML technology, the new bar will be set by the first few institutions that can effectively identify criminals with a systemic approach, meaning people and technology, and who do it to a superior level. If a financial institution is late to the party, it is going to find itself compared to others who are doing a much better job because they are deploying newer technologies. A solution like the QuantaVerse Platform is going to be a competitive technology simply because it's going to reduce the cost of transactions, which is top of mind for financial institutions.
Everyone is looking to reduce the friction in the system or reduce some of the costs related to global transactions. Financial institutions who are late to the party will have expensive and slow, error-prone operations with either a lot of false positives or the inability to identify and catch criminals. Not keeping up with more progressive financial institutions will result in either high penalties or high operating costs through false positives.
-
Leaders Must Become More Technology Savvy
AI algorithms evolve because they detect patterns, which they then deliver to those who can build on those patterns. Because the human mind can understand so many more vectors than a machine can, it makes sense that the future compliance officer in the AML space is not so much about watching flows go through an AML department, figuring out false positives and clearing workloads before the close of business. Rather, it'll be much more about helping understand and evolve pattern recognition for their institution.
Criminals tend to operate in patterns. They often use the same types of addresses and same types of shell companies. Leaders on the business side really need to understand the systems they’re using and what goes into them. And on the IT side, they must implement in such a way that the system continues to evolve and stays a few paces ahead of the bad guys.
-
Buy Before Building
Agile development and SaaS delivery models have made buying solutions more prevalent than ever. When it comes to AML technology and data, the best advice is “buy before building.” Building requires a team of data scientists and other specialists to be on staff. The costs are high, and the returns do not pay off - especially given building AML solutions is probably not within your institution's core skillset. SaaS models make it easier than ever to connect your database, and get on with the business of stopping the bad guys.
-
Cost Metrics and Operational Resiliency Matter
For many institutions, regulator findings, big penalties, or bad transactions that slipped through cracks become wake-up calls to look at their costs more closely. By then it's often too late. Instead of being alarmed by the wake-up calls, financial institutions should be eager to drive down their component cost of a transaction, their unit cost to process a transaction, and reduce the number of false positives they must address.
The most sophisticated goal, which many institutions may not currently be able to determine, is the component cost of a transaction cost. For example, of a payment cost, what percentage of the cost arises from of AML operations? Determining this provides the component cost - the amount charged per payment within an institution to provide a service to make sure the payment stream is clean.
Other numbers worth paying attention to include the number of false positives driving up the operating expenses, which in turn drives up operating risk, and human error, which drives up misses. Then there’s the big number, the criminals that weren’t caught. You don’t generally catch them until an external entity figures out that you didn't catch them. And then you face steep penalties.
Operational resiliency is another area to be considered. It’s very hard to assemble and manage hundreds and hundreds of people in multiple locations to work on the back end of a TMS. Given that the job is very challenging, there is also the issue of keeping people focused and motivated. Financial institutions also face data privacy and data security issues if they want investigative staff to work remotely. Many of these problems would be easier to handle with a high degree of automation and a lower human factor.
The QuantaVerse Platform leverages the latest and most powerful AI, machine learning, and data analytics tools and trends to help companies manage financial crime risks more effectively and affordably. Doing so addresses three major AML issues – reducing false positives, automating investigative steps, and identifying risk that legacy systems regularly miss; making it fast, easy, and cost effective for financial institutions to benefit from the latest AML technology.