3 min read
How to Meet New Regulatory Demands for AML Innovation While Ensuring Transparency
AML RightSource
:
January 18, 2022
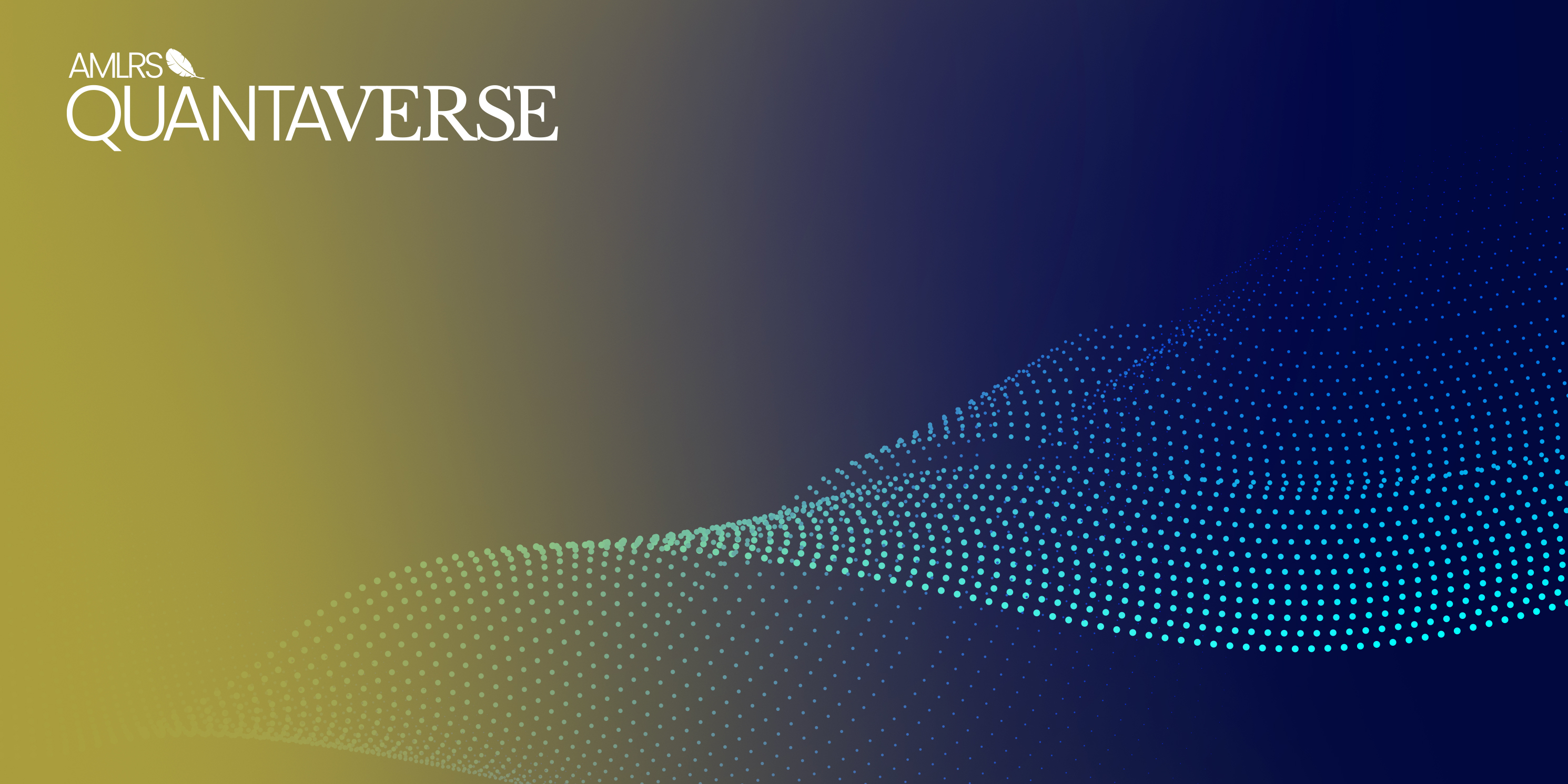
Watch this video to learn more about the QuantaVerse Platform
A key part of the role of Chief Compliance Officer is satisfying both regulatory guidelines and the regulators that monitor their programs. That has meant staying the course with an AML program that checked regulatory boxes even if outcomes were less than effective. Consider that the industry has operated knowing that an estimated 80% of dirty money being laundered through global financial institutions goes unidentified.
This reality has never satisfied those who have dedicated themselves to a career finding and eliminating criminal and terrorist money laundering. Compliance teams want to do more than check regulatory boxes; they are focused on reducing the financial and professional risk associated with letting financial crime infiltrate their institution. Regulator examiners want to see AML programs be effective instead of just compliant.
Recent legislative and regulatory action around the globe signal that less-than-effective AML programs are no longer acceptable. The advanced technology and innovation that compliance leaders know could improve AML/BSA programs, but worried might be a concern to their regulators, is now expected.
Regulators Expect Innovation
In summer 2021, a special report from the European Court of Auditors disclosed that EU banking efforts to fight money laundering “were fragmented” and “implementation is insufficient.” These finding were the foundation of calls to establish an EU Anti-Money Laundering Authority (AMLA) which would help to ensure EU AML and countering the financing of terrorism (CFT) rules were “correctly and consistently” applied.
By late July, the European Commission presented an ambitious package of legislative proposals to strengthen the EU’s AML and CFT rules. These measures greatly enhance the existing EU framework by considering new and emerging challenges linked to technological innovation.
The January 2021 passage of the AML Act in the U.S. included clear expectations for innovation and measurable improvement in program efficacy. Specifically, the act called for:
- U.S. government promotion of technological innovation for AML and CTF purposes
- Authorities must adapt to changing conditions and to innovations in AML/CTF
- FinCEN and federal functional regulators to appoint “innovation officers” to make institutions and other stakeholders aware of new methods and technologies that may assist in compliance.
Deploying AML Innovation
With regulatory agencies and financial institution leaders agreeing that innovation and automation offer a crucial path to improvement, it is time to modernize AML/BSA compliance technology, systems, and practices. Institutions are now testing and deploying advanced technology as part of their AML compliance program including artificial intelligence (AI) and machine learning. Fortunately, some AI-enabled AML compliance solutions are designed to seamlessly work with existing systems keeping start-up costs low. The right solutions also enable institutions to do more with the AML teams they have, even as demands grow.
Learnings from financial institutions that have already applied AI innovations to their AML/CFT efforts are roadmaps for other institutions. Recommendations on implementing AML innovations from Chief Compliance Officers who have already done so include:
- Document Your Situation - Know your number of cases investigated, estimate the time spent on types of cases, calculate associated costs (internal as well as outsourced), rank your confidence in terms of accuracy and consistency.
- Dig in on Vendor Selection - Legacy TMS providers give lip service to innovation, while AI companies may not have the expertise and focus required to address specific AML needs. Some AI-enabled AML solutions address only one or a few steps of the investigative process (i.e., adverse media or entity resolution) while more sophisticated systems automate all steps of the investigative process.
- Test by Executing a Discrete Part of your Program - For example, testing a technology on high-risk entity reviews will show you what is possible and how a new technology works with your existing systems and team. The right vendor should be able to give you test results in a matter of weeks.
- Get Senior Level Buy-In - Share results of program enhancement and testing with senior risk and compliance executives.
- Develop a Comprehensive Roadmap - Focus on one part of your AML program at a time – start with automating investigations, then false positive reduction, and ultimately find the risk from false negatives that current systems are missing. The right software vendor can address all these critical aspects of your AML/BSA compliance program.
Maintaining Transparency
Regulatory demands for AML innovation don’t reduce the requirement for decision-related documentation and reporting. Done without insight into AML requirements, an AI solution could create a “black box” scenario, but a well-designed machine learning solution can provide the same level of abstraction as a human investigator might when clearing a case or documenting a SAR.
For example, in the QuantaVerse Financial Crime Investigation Report (FCIR), AI decisions are accompanied by explanations of the type of regulatory violations, the scope of a suspicious behavior, and confidence level of risk analysis.
One way the QuantaVerse decision engine explains individual predictions created by its machine learning models by using what data scientists call “Local Interpretable Model-Agnostic Explanations.” You can call it “LIME.” LIME creates surrogate models that are easily understood abstractions of the hundreds of features and thousands of parameters the actual models consider.
An everyday analogy is a system developed to support better medical diagnoses. The model receives a long list of current symptoms along with the patient’s demographics, health history, medications, persistent conditions, etc. All this data is considered by the model which predicts that the patient has the flu. The LIME explainer parses from myriad data only those observables that were essential to the flu diagnosis – sneezing, headache, and fatigue. A doctor reviews the explainer model and makes a final determination. Just like an AML investigator, a doctor can dig further into the data if they feel they must before rendering a decision.
Learn more about the QuantaVerse Platform’s AI-powered AML solutions and how you can easily make new innovations part of your AML compliance program at: https://quantaverse.net/try-ai.