3 min read
Machine Learning and Robotic Process Automation: the Next Steps in AML Technology
Ruben Aguero Quinteros
:
October 25, 2019
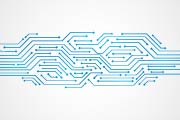
Since the passage of the Bank Secrecy Act in 1970, FIs have looked for ways to automate elements of their AML compliance programs. Early automation included integration of exemption lists with teller systems, automatic printing of CTRs, accumulation of CTR information for bulk filing, integrating OFAC list screening with transaction processing systems and use of rules based systems to identify potentially suspicious transactions. More recently with the broadening of FI delivery channels, the rising cost of meeting regulatory mandates and the increasing maturity of Artificial Intelligence (AI), FIs are exploring (and in some cases deploying) new tools to enhance their AML compliance efforts.
AI broadly refers to computational algorithms which are programmed to improve their performance based on experience, mirroring to a certain extent the manner in which humans learn to make decisions. One subset of AI is Machine Learning (ML), an automated model building method which works based on data analysis and pattern recognition as opposed to being explicitly programmed with instructions. ML differs from conventional computational algorithms in that it is not explicitly programmed to perform a set of tasks, but rather to use inference and pattern analysis to make decisions.
The “learning” in ML is accomplished by feeding the algorithm training data - a set of inputs and corresponding outputs - that the program processes. The program automatically adjusts its parameters to fit the training data, thus creating a generalization as an output. The performance of this generalized model is then compared against test data - inputs which are new and previously unseen by the algorithm.
ML in the AML industry has transitioned from exploration to adoption. This progress is the result of collaboration between AML experts and data scientists to adapt ML to industry needs. In deploying ML, users need to decide whether to maintain legacy AML systems and enhance them with ML algorithms or replace them completely. Early adopters are trending toward using ML as an enhancement, allowing them to rethink existing processes, such as reducing AML legacy system’s false-positives or identification of new risk segments and patterns. This involves feeding emerging financial crime typologies into the systems; such ML algorithms can stay abreast of the new illicit patterns, providing faster adaptation than programming monitoring rules and tuning them.
Another technology which can support AML compliance is Robotic Process Automation (RPA). RPA simulates mouse and keyboard commands to automate human interaction with software Graphical User Interfaces. This allows business processes which are repetitive and have a set, logical process flow to be automated through mouse and keyboard navigation with additional controls such as pixel recognition, Optical Character Recognition, time delays, etc. Use of RPA can lead to greater service quality, exceptional ROI, increased process automation, improved compliance, greater business agility, and improved total business value[1].
Deployment of RPA in the AML industry is just beginning. The slow pace of adoption stems in part from a lack of understanding of the technological solution alternatives and what areas they can best be used for in AML compliance operations and from the speed at which solution vendors are adding the functionality to in place systems.
In a December 2018 joint statement regarding innovative technologies, FinCEN and other federal agencies welcomed “these types of innovative approaches to further efforts to protect the financial system against illicit financial activity”[2]. This regulatory guidance should help RPA attain increased levels of integration given the potential benefits it brings to the AML industry.
ML is an evolving tool for assisting with more strategic activities such as customer segmentation for risk management and tuning and optimization exercises; it can help FIs reduce costs by targeting false-positives and creating more granular alerts for suspicious activity reporting. RPA can be useful for automating repetitive data-gathering processes performed prior to a subjective task; it cuts down on costs by reducing the amount of data collection and summarizing an analyst has to do before dispositioning an investigation. In the near term, the availability and facility of ML- and RPA-enhanced workflows will have a positive impact of the effectiveness of AML compliance at adopting institutions.
How AML RightSource can help
Our Financial Crimes Advisory practice has assisted numerous institutions in software vendor selection and implementation in a project management capacity. In addition, our Managed Services team of BSA / AML analysts is ready to be deployed in RPA- or ML-enhanced production workflows.
[1] https://www.aaajournals.org/doi/full/10.2308/jeta-10589#n3
[2] https://www.fincen.gov/sites/default/files/2018-12/Joint%20Statement%20on%20Innovation%20Statement%20%28Final%2011-30-18%29_508.pdf