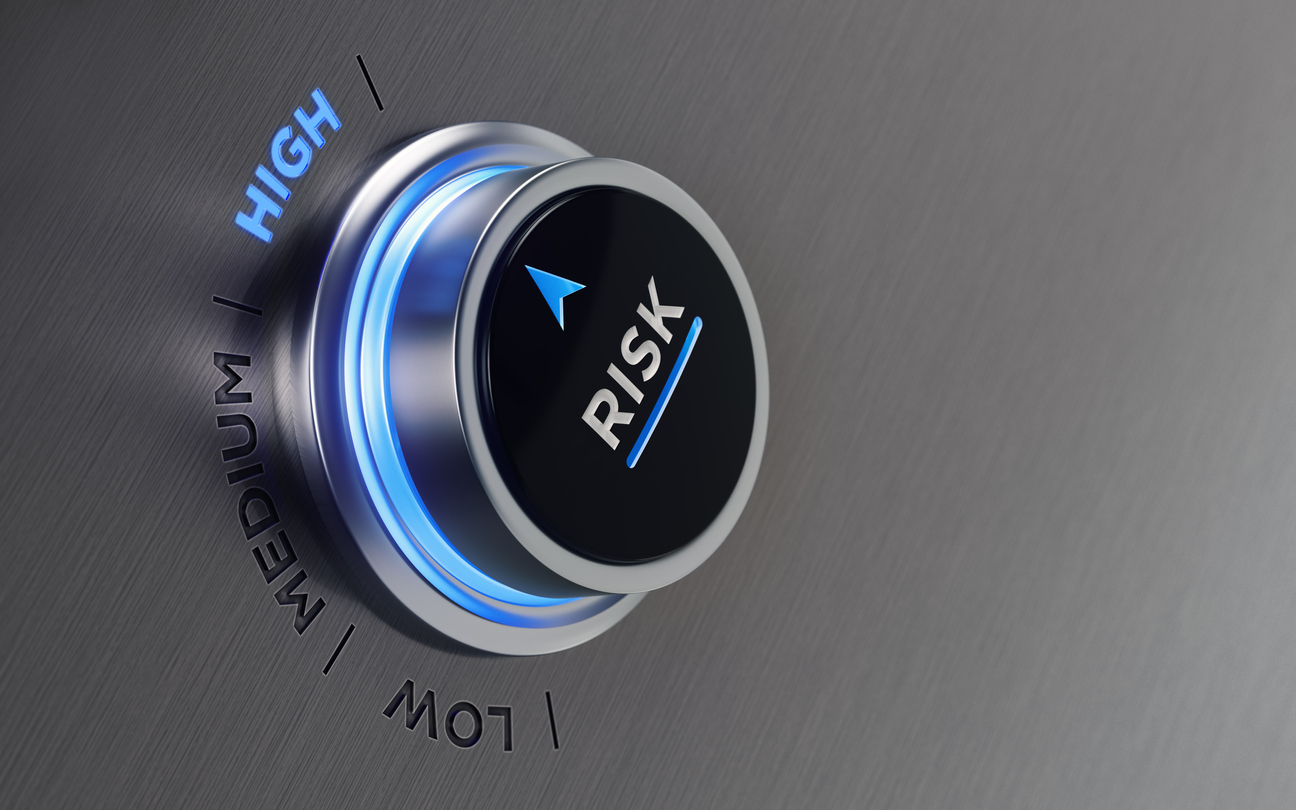
While planning for a panel on Enhanced Due Diligence (EDD) for high risk clients, I was provided with pre-conference questions submitted by attendees. One of them started with “All MSBs are high risk…”, and another with, “Since all non-profits are high risk…”. My immediate reaction to both was to wince for a number of reasons, including that it is simply not true and can have the dramatic effect of preventing financial access to those entities and cause unnecessary economic harm.
As Anti-Money Laundering (AML) Professionals, we sometimes get complacent and fall back on conservative stances when it comes to high risk clients. It is easier on some level to paint customer types with a wide brush and treat an entire group as high risk, rather than take the time to make more granular or tiered assessments of risk. While it may take a bit more time up front, the benefits in future time saved can outweigh the investment.
The intent of the Bank Secrecy Act is for financial institutions (FI) to assist law enforcement by detecting and reporting suspicious activity. Law enforcement does not care what the FIs risk rating of the SAR’d customer is, they just want intelligence to assist with starting and furthering their investigations. It feels like we have lost track of this on some level and become too wrapped up in identifying high risk clients just for the sake of identifying high risk clients. The broad swaths of client types identified in the FFIEC manual are being taken too literally; bogging FIs down with manual enhanced due diligence (EDD) reviews of clients in particular business types or with particular affiliations.
As an example, Senior Foreign Political Figures (SFPF) are many times considered by financial institutions as high risk, while discounting the controls applicable to the particular individual. It’s no shock when these SFPFs start with high inherent risk regardless of the mitigants, and then retain a residual rating of high. SFPFs, like any other group of potentially high risk clients, should be considered on a case-by-case basis and open to any risk level in the client risk rating hierarchy.
SFPFs come in many flavors and fall on a spectrum of risk. The most risky would have at least 3 of the following factors:
- holds a material position in a foreign government that has lax financial monitoring controls
- holds a position in a foreign government that allows them access to public funds
- holds a position that has little oversight and/or can act unilaterally
- is associated with known bad actors
- has been associated with corruption
- has the intent to take or earmark public funds for personal gain.
Some of these factors such as intent are normally unknowable but the others should be reasonably easy to identify. Without a significant combination of the above factors, an SFPF may be completely benign, and would not warrant the time and effort of a high risk designation.
A robust framework containing onboarding guidelines for prohibited customer types, restricted customer types and potentially high risk customer types coupled with a weighted customer scoring tree, should identify the lion’s share of potentially high risk customers at or before account opening. Potentially high risk customers that slip through the net are likely misrepresenting themselves or at least withholding information that would affect their risk profile.
A well-tuned, risk-based Anti-Money Laundering monitoring system should be able to identify potentially high risk customers that bypass the onboarding net based on volume, velocity, historical behavior variance, or significant divergence from peers.
Regardless of how they are identified, SFPFs should be investigated based on the outcome risk assessed at an appropriate level. Base this determination on all factors and mitigants present, rather than checking a particular box and letting that indicate risk.
A future state enhancement should include an application of machine learning to more accurately identify customers with a likelihood of a Suspicious Activity Report (SAR) filing. Rather than focusing on static attributes and activity thresholds to identify high risk clients, we should start with clients on whom we have filed a SAR and reverse engineer a set of factors and parameters that would lead us to other clients that have similar attributes collected at account onboarding, or during the life of the account. Some of this is already being done to tune scenarios in Transaction Monitoring (TM) systems but it should also be done for the high risk client space.
Moving the dial from a 98% to 97% false positive rate in the TM system via machine learning can seem huge, but the impact on staffing and risk can be minimal. Reducing the number of high risk clients while also better identifying clients with an actual propensity for SAR filing, could benefit both staffing and the risk rating of an FIs customer base in a meaningful way.