4 min read
Part 3: The Role of Large Language Models in Modern AML
Rachel Dettmer
:
November 01, 2023
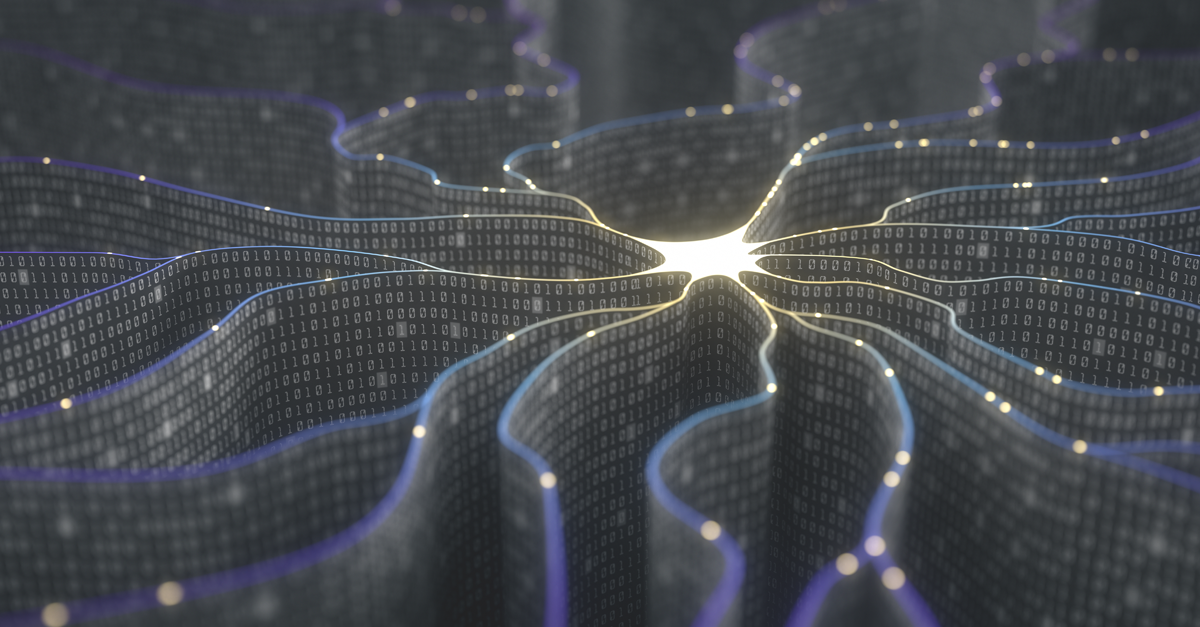
In the intricate landscape of contemporary financial systems, banks and financial service providers must dynamically adapt to mitigate risks and capitalize on business opportunities.
The integration of Artificial Intelligence into Anti-Money Laundering (AML) frameworks in recent years has revolutionized the methods employed by institutions in their efforts to combat financial crime.
As more advanced Large Language Models (LLMs) like ChatGPT and LLaMA emerge as mighty AI tools, their role in shaping the future of AML strategies across the globe will only become more significant.
What are Large Language Models?
A large language model is a type of artificial intelligence designed to process, understand, and generate text (cue generative AI).
In contrast to other AI models that require handcrafted rules or specialized architectures for each task, they can perform a wide variety of natural language processing tasks with minimal task-specific training.
How do they do this? Well, it’s a combination of sophisticated and clever techniques.
First, developers found a way to bypass training a model with inputs and outputs by using vast amounts of text scraped from open sources on the web, minimizing the time and cost normally involved.
Next, the models typically use transformers, a type of neural network architecture designed to process sequential input data. This allows them to process all words or tokens in parallel for faster performance and the attention mechanism also found in this architecture allows the model to focus on the most relevant parts of the input for each output.
As a result, these models are highly adaptive and demonstrate remarkable generalization capabilities.
With this ability to comprehend context, generate human-like responses, perform translation, and discern patterns across millions of documents with ease – they have become indispensable tools.
What are the benefits of Large Language Models in AML?
These models have the power to transform conventional approaches to investigating and combating financial crime by refining the process, increasing productivity, and reducing costs.
Refined AML Processes
LLMs offer a more efficient and accurate approach to detecting suspicious activities by:
- Augmented Risk Assessment: When functional or quality gaps are identified during an assessment, such as search or analysis, two key areas of compliance, LLMs can be employed to manage these areas more efficiently in comparison to manual processes. This expeditiousness and precision can help institutions prioritize high-risk cases requiring immediate attention and speed up the process from identifying suspicious activity to filing financial crime reports (FCRs).
- Regulatory Compliance: By remaining current on the latest regulations and best practices, these models can facilitate adherence to regulatory compliance more effectively. They can also streamline reporting processes by automating the generation of required documentation.
- Global Expertise: With their capacity to process information from various sources in multiple languages, LLMs can assist organizations in traversing intricate international rules and regulations.
Productivity Gain by Financial Investigators
These models offer significant productivity gains for financial investigators by:
- Streamlining Research: Investigators can utilize AI-generated summaries or reports from LLMs to quickly glean insights into a specific case or trend without devoting hours to manual research.
- Automating Routine Tasks: LLMs can automate tasks such as data entry, document sorting, or initial assessments, liberating investigators' time for more critical tasks.
- Enhancing Collaboration: By providing real-time access to pertinent information across teams or departments, LLMs facilitate improved collaboration among investigators. Furthermore, they empower non-technical personnel to engage with complex datasets through natural language queries, fostering greater collaboration among investigators and management.
- Freedom from Complex User Interfaces: LLMs facilitate quicker response times between detecting suspicious activities and submitting FCRs by enabling researchers to interact with data using natural language.
Cost Savings for Financial Institutions
In addition to enhanced risk assessment capabilities and compliance support, LLMs contribute significantly to cost savings for financial institutions. Here are three examples:
- Scalability and ROI: LLMs are catalysts for growth. They can effortlessly scale up, adapt to evolving needs, and efficiently handle increased workloads within the AML workflow, generating and sustaining value for organizations.
- Lower Operational Expenses: Automation curtails the time spent on manual processes and diminishes overall operational costs associated with AML efforts.
- Minimized Regulatory Fines: Superior risk assessment capabilities result in fewer regulatory violations that could lead to substantial fines.
The Synergy Between Large Language Models and Human Investigators
While LLMs offer impressive capabilities, they are not intended to displace human investigators. The human language is only the tip of the communication iceberg, and these models work on highly mathematical probabilities.
Instead, they provide investigators with the most powerful tools to perform their jobs faster, more efficiently, and more consistently.
Banks often face backlogs with thousands of cases and alerts, creating a daunting and expensive challenge for compliance teams.
The integration of LLMs with human expertise can enable investigators to tackle this ubiquitous and ever-increasing backlog problem without compromising on accuracy or quality.
The key lies in harnessing the complementary strengths of both AI-driven models and human investigators to create a robust AML framework.
These models excel at automating routine tasks, processing vast quantities of data, and identifying complex patterns. Meanwhile, human investigators bring nuanced understanding, adaptability, and critical thinking skills that allow them to interpret intricate situations and make informed decisions when dealing with suspicious activities.
By combining these strengths, organizations can create a synergistic relationship between LLMs and human investigators that not only addresses the backlog issue but also strengthens overall AML efforts.
Limitations of Large Language Models in AML
Despite their potential benefits, there are limitations associated with utilizing LLMs in the context of AML.
To function effectively, these models necessitate access to vast quantities of sensitive information, and there are concerns over data privacy, consent, and security. Likewise, they rely heavily on accurate and comprehensive data for optimal performance and to exclude any bias.
There are also some ethical and legal considerations surrounding the use of AI-generated content.
LLMs often lack transparency and interpretability, making it difficult to understand how they generate responses. They also require little human interaction. When it comes to decision-making purposes in compliance activities, this could be problematic.
So, how can we safely benefit from the power of LLMs when integrating them into AML processes despite their limitations?
Constructing a Resilient AML Strategy with LLMs
Financial institutions must strike a balance between employing AI-driven tools and human expertise to devise a comprehensive strategy that ensures regulatory compliance while mitigating risk.
It is important to be aware of inherent weak points and use rigorous evaluation and probing methods to target them in specific use cases.
Proper data protection measures and the quality of input data are crucial and essential for achieving reliable results.
While they can automate numerous tasks in AML compliance efforts, there will always be a need for human expertise in interpreting complex situations or suspicious activities, and it should never be a question of replacing humans. Instead, it should be one of ‘how can we enhance what our people are doing.’
By integrating cutting-edge technology such as GPT or LLaMa with traditional managed services offerings and proficient personnel, organizations can construct resilient anti-money laundering strategies that adapt rapidly to fluctuating regulatory environments while reducing costs associated with non-compliance.
Want to hear more? Listen to our podcast, 'Exploring Large Language Models' here!
Or, if you want to find out more about how we currently use the latest and finest technology to fight financial crime, fill out our contact form, and let’s start the conversation.